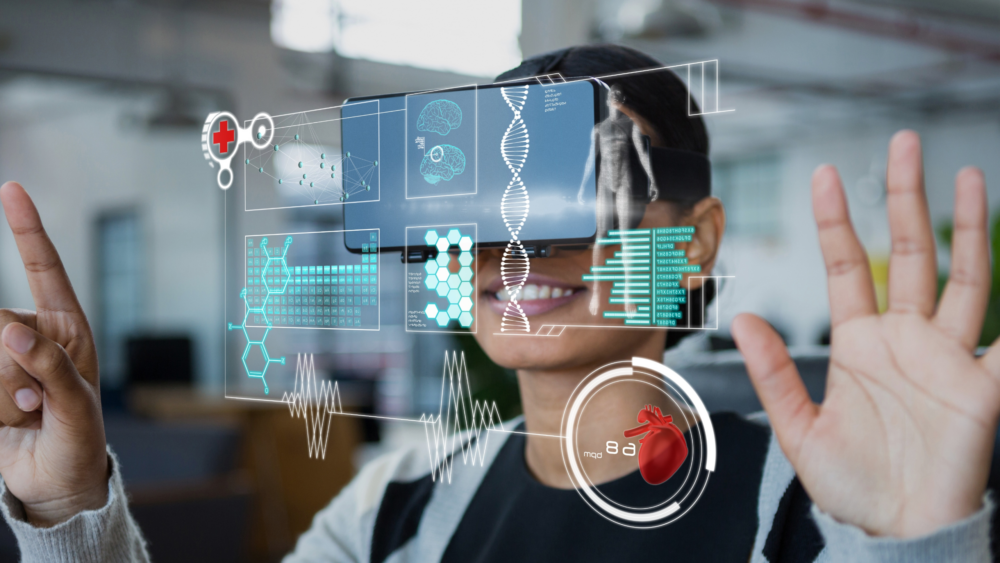
The increased availability and variety of real-world data (RWD) has created new drug development and discovery opportunities. Its systematic use has increased the understanding of medicinal products safety, efficacy, and utilization in real-world settings. Good quality RWD will be crucial to improve patient outcomes by providing insights that complement traditional clinical trial data. But, as data complexity grows, the challenges that could limit the usefulness of RWD are magnified. Amongst these, questions around relevance, depth and quality of the source data, data privacy and access, need to be resolved. Also, agreement on appropriate analytical methods must be seeked. In this blog, we will explore what constitutes good quality RWD, and highlight its importance for the drug development and discovery process.
Defining Good Quality Real-World Data:
Although there is not a formal definition of Good Quality RWD, it is in general understood that they are the data that are collected from routine clinical practice, reflecting real-world patient experiences and outcomes. However, their quality must be critically assessed to determine if they are suitable to use. This means that be considered good quality, data need to meet several key criteria:
Accuracy: RWD should be reliable and free from errors and biases. It should be collected using standardized methodologies to ensure consistency and precision.
Completeness: The data should encompass a wide range of relevant variables, including patient demographics, medical history, treatment patterns, clinical outcomes, and healthcare resource utilization. Comprehensive and complete datasets provide a holistic view of patient populations.
Representativeness: RWD should accurately represent diverse patient populations, including different demographics, comorbidities, and socioeconomic backgrounds. Representative datasets allow for generalizability of findings to real-world clinical practice.
Data Source Reliability: The source of RWD, such as electronic health records, claims databases, or patient registries, should have strong data governance mechanisms and validation processes in place to ensure data integrity.
Given the different potential uses of good quality RWD, data quality standards and best practices may vary across countries, regulatory agencies, and research organizations. In many pharmaceutical organizations, Data Landscape teams have become the de facto responsibles for managing and optimizing RWD collection, integration, and analysis. Yet, the continuous improvement of good quality RWD will require close collaboration among stakeholders, including healthcare providers, researchers, regulators, and industry professionals.
Using Good Quality RWD in Drug Development and Discovery
As the pharmaceutical industry faces increasing demands to deliver drugs with improved patient outcomes in a cost-containment, highly scrutinized scenario, using good quality RWD will be of paramount importance. Some of the key applications of good quality RWD include:
Preclinical Research: RWD can inform preclinical research by identifying unmet medical needs, understanding disease progression, and identifying potential therapeutic targets. It provides valuable context for selecting appropriate drug candidates for further development.
Clinical Trial Design and Recruitment: RWD can aid in optimizing clinical trial design by informing patient selection criteria, identifying appropriate control groups, and refining outcome measures. It helps ensure that clinical trials reflect real-world patient populations, enhancing external validity.
Safety and Efficacy Assessment: Good quality RWD provides a robust evidence base for assessing the safety and effectiveness of medications beyond controlled clinical trial settings. It helps monitor post-approval safety signals, evaluate long-term outcomes, and detect rare adverse events.
Comparative Effectiveness Research: RWD allows for comparative effectiveness research, enabling comparisons between different treatment options in real-world clinical practice. It helps identify optimal therapies, refine treatment guidelines, and support shared decision-making between patients and healthcare providers.
Regulatory Submissions: RWD can supplement data from clinical trials by providing long-term follow-up data, evaluating outcomes in specific patient subgroups, assessing real-world treatment patterns, and examining comparative effectiveness against standard of care or alternative therapies.
Regulatory and market examples:
Overall, good quality RWD serves as a valuable resource for regulatory bodies, pharmaceutical companies, payers, and healthcare providers, enabling them to make informed decisions, improve patient outcomes, and optimize healthcare delivery. Numerous studies have demonstrated the value of good quality RWD in drug development and discovery:
The FDA’s Sentinel Initiative: The FDA’s Sentinel Initiative utilizes RWD from various sources to monitor the safety and effectiveness of medical products. It has facilitated the identification of safety concerns and supported regulatory decision-making processes.
The use of RWD in oncology: RWD has been instrumental in assessing the safety and effectiveness of cancer therapies, supporting label expansions, and generating real-world evidence. Studies have shown the impact of RWD in identifying patient subgroups who may benefit from specific treatments.
RWD in rare diseases: Good quality RWD has been particularly valuable in rare diseases, where clinical trial data may be limited. It has helped understand disease progression, natural history, and treatment patterns, informing drug development strategies and patient care.
Good quality RWD is a powerful asset in the drug development life cycle, offering invaluable insights into real-world patient experiences, safety, and effectiveness of medications.
In conclusion, incorporating good quality RWD into drug development processes has transformative potential for the pharmaceutical industry. It enables a deeper understanding of drug performance in real-world settings, enhances patient safety surveillance, and supports evidence-based decision-making. By ensuring the quality of RWD, researchers and drug developers can improve the effectiveness, safety, and value of pharmaceutical interventions, ultimately benefiting patients and healthcare systems worldwide.
Sources:
Real-World Data Quality: What are the Opportunities and Challenges? by McKinsey. This article discusses the quality of RWD, focusing on the challenges and opportunities associated with ensuring the quality of RWD. It also provides recommendations for improving the quality of RWD. [Link: https://www.mckinsey.com/industries/life-sciences/our-insights/real-world-data-quality-what-are-the-opportunities-and-challenges]
Real-World Data: A Brief Review of the Methods, Applications, Challenges and Opportunities by BMC Medical Research Methodology. This article provides a brief review of the methods, applications, challenges, and opportunities associated with real-world data. It also discusses the importance of ensuring the quality of RWD. [Link: https://bmcmedresmethodol.biomedcentral.com/articles/10.1186]
EFPIA’s Position on the Use & Acceptance of Real World Evidence by International Markets https://www.efpia.eu/media/602956/efpia-rwe-position-paper_aug2021.pdf A must read position paper on EFPIA’s view on the use and acceptance of Real World Evidence (RWE) to support benefit/risk decision-making.
The Regulatory road to Innovation by EFPIA https://efpia.eu/media/541132/efpia_regulatory-road-to-innovation_leaflet.pdf An infographic about EFPIA’s vision of future drug development and discovery
Klimek P et al Quality Criteria for Real-world Data in Pharmaceutical Research and Health Care Decision-making: Austrian Expert Consensus. JMIR Med Inform. 2022 Jun 17;10(6):e34204. doi: 10.2196/34204. PMID: 35713954; PMCID: PMC9250059.
Valla V et al Use of Real-World Evidence for International Regulatory Decision Making in Medical Devices. International Journal of Digital Health DOI: 10.29337/ijdh.50